New Research, On the Generalization of Deep Learning Models for AoA Estimation in Bluetooth Indoor Scenarios, by Ivan Pisa, Guillem Boquet, Xavier Vilajosana, and Borja Martinez of Universitat Oberta de Catalunya, Barcelona, Spain, looks into the application of Deep Learning (DL) models for Angle-of-Arrival (AoA) estimation, a key technique for indoor positioning in Bluetooth indoor positioning.
Accurate estimation of the Angle-of-Arrival (AoA) is complex. The accuracy of AoA estimates can be significantly affected by various signal disturbances such as multipath components, polarisation, spread delays, jitter and noise. These factors can create ambiguities and distort the phase differences of received signals, leading to errors in the position data reported by the system. Also, the multipath effect, where multiple signal replicas interfere with each other, can severely mislead AoA estimations.
Conventional algorithmic AoA estimation techniques rely heavily on processes that can increase the cost, reduce scalability and complicate the operation of the systems where they are used. A primary requirement is the calibration of the antenna array to obtain its steering vector, a process that ensures accurate directional sensitivity of the antenna system. This calibration, along with other computationally intensive tasks such as matrix inversion and eigenvector decomposition, requires significant computational resources. This can be particularly challenging when these systems need to be scaled up for large deployments.
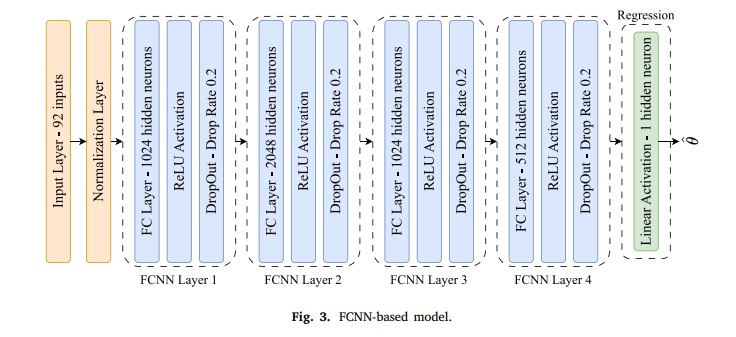
The study’s main objective was to evaluate and compare the generalisation capabilities of AI machine learning models to traditional signal processing techniques, such as the Multiple Signal Classification (MUSIC) algorithm, across various scenarios including different locator positions, time instant and unfamiliar environments.
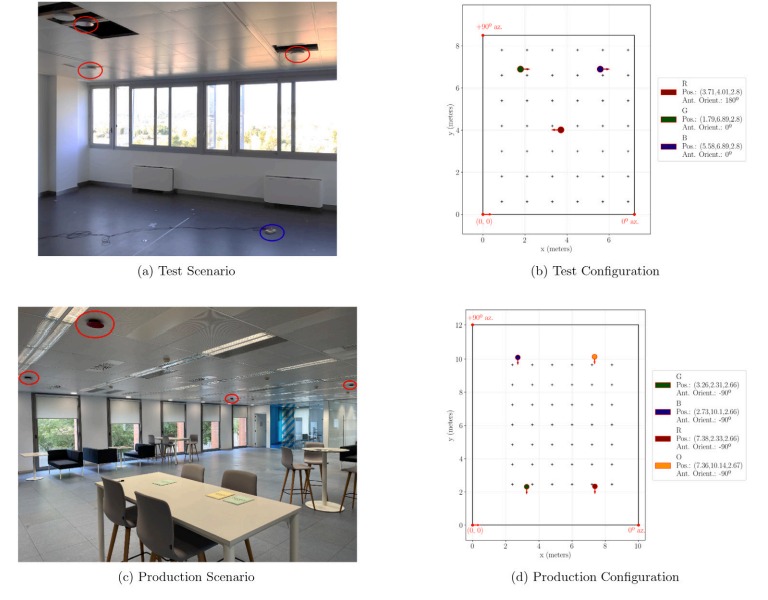
The results indicated that while DL models perform well within the environment they are trained in, their ability to generalise to new or altered conditions is notably weaker than that of the MUSIC algorithm. The authors concluded that DL models tend to learn specifics of the training environment rather than generalisable features of the AoA estimation task. This learning limitation hampers their practical application since models trained in one environment perform poorly in another.