There’s a new article from the Icontech International Journal of Surveys, Engineering, Technology on Indoor Position Routing (IPR) and Data Monitor Using Bluetooth Low Energy Technology by researchers at the Hasan Kalyoncu University, Institute of Science, Electrical & Electronics Engineering, Gaziantep, Turkey.
This article is different because it considers navigation as opposed to just locating. It explains the advantages of Bluetooth LE over WiFi and also compares with RFID:
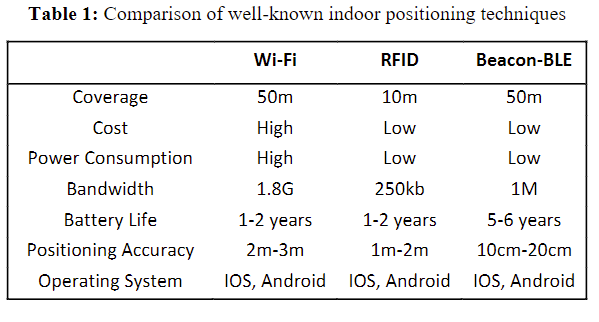
Trilateration, Received Signal Strength Indicator (RSSI) and Decibel-milliwatts (dBmW) are explained and how these fit into locating position.
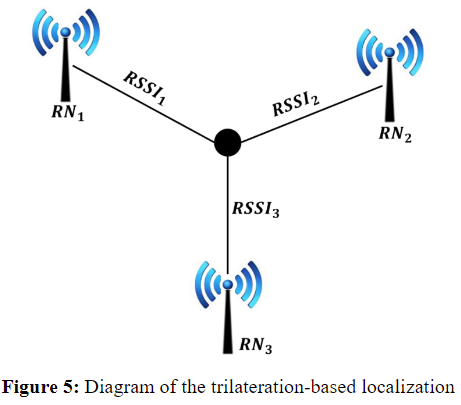
The article describes a system created for navigation that uses iBeacon sensor nodes, an Android device and app.
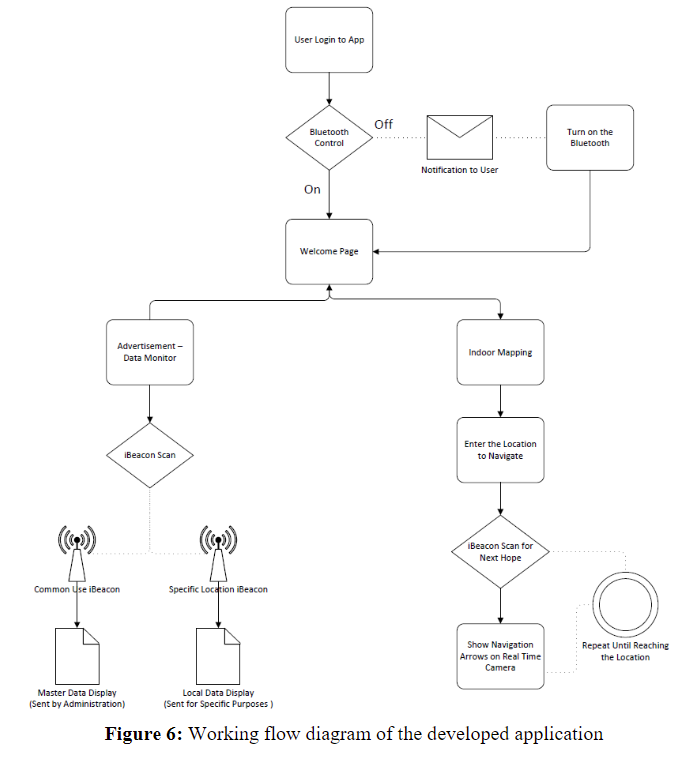
Read Determining Location Using Bluetooth Beacons
Read Using Beacons, iBeacons for Real-time Locating Systems (RTLS)