There’s a recent research paper on Indexing for Moving Objects in Multi-Floor Indoor Spaces That Supports Complex Semantic Queries. It says humans spend 87% of their time in indoor spaces such as private residences and office buildings and it’s becoming more important to be able to derive meaning from indoor location.
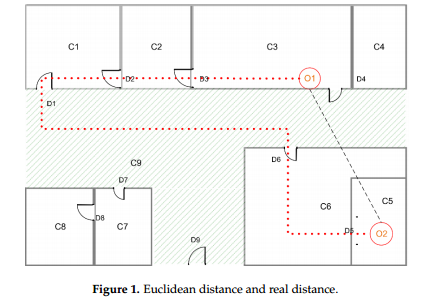
The paper explains how outdoor moving object management technology, which is very mature, cannot be applied to indoor spaces. Instead you need software that not only understands floors but also multi-floors and inter-floor (elevators and stairs) cells. The paper describes an index that can store indoor moving objects in multi-floor indoor spaces that can support 3D spatial queries.