There’s new research evaluating the performance of a Bluetooth Low-Energy (BLE) positioning and direction-finding system under conditions that closely mimic real-world usage. The aim of the study was to enhance a BLE-based hybrid algorithm, which integrates both positioning and direction-finding capabilities. The researchers focused on evaluating the system in realistic conditions, which included using multiple types of devices, separating the devices used for creating the database from those used for evaluation, and ensuring a sufficient time gap between data collection and evaluation measurements.
The hybrid algorithm used in the study combines proximity detection, based on the strongest Received Signal Strength Indicator (RSSI), with a fingerprinting approach, where the evaluation data is compared to a pre-existing database. By limiting the search area for positioning to locations with the highest RSSI, the algorithm aims to reduce significant positioning errors. The study also integrated direction-finding functionality into the algorithm, taking into account issues such as signal obstruction caused by the user’s body, which can block radio signals from certain directions.
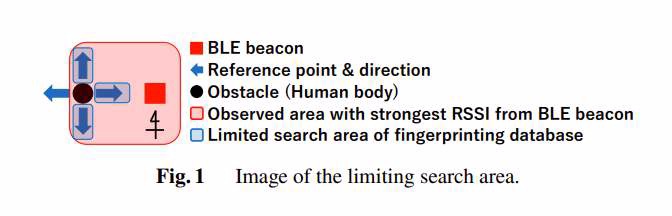
The evaluation was conducted in a corridor environment, with BLE beacons installed along the walls and ceilings. The research utilised five different smartphone models for both data collection and evaluation. To simulate real usage, measurements were taken from four directions at each evaluation point. The study compared the performance of this hybrid method with a previously proposed method that only included direction estimation based on signal divergence.
The findings demonstrated that the hybrid algorithm significantly outperformed the earlier method in terms of both positioning and direction-finding accuracy, especially under realistic usage conditions. Although the performance of the system declined when the intervals between the BLE beacons were increased, it remained at an acceptable level even with fewer beacons installed. This suggests that the hybrid algorithm is robust and effective, even when the system’s infrastructure is reduced.
In conclusion, the study demonstrated the effectiveness of the hybrid BLE algorithm for positioning and direction-finding in realistic environments. The findings emphasised the importance of conducting performance evaluations under real-world conditions, which better reflect the challenges and variability of actual usage.