Researchers from Department of Computer Science, University of Jaén, Spain have a new paper on OBLEA: A New Methodology to Optimise Bluetooth Low Energy Anchors in Multi-occupancy Location Systems.
This paper introduces a new methodology called OBLEA, which aims to optimise BLE anchor configurations in indoor settings. It takes into account various BLE variables to enhance flexibility and applicability to different environments. The method uses a data-driven approach, aiming to obtain the best configuration with as few anchors as possible.
The OBLEA method offers a flexible framework for indoor spaces where the occupants are fitted with wrist activity bracelets (beacons) and BLE anchors are set up. The anchors then collect and aggregate data, sending it to a central point (fog node) via MQTT.
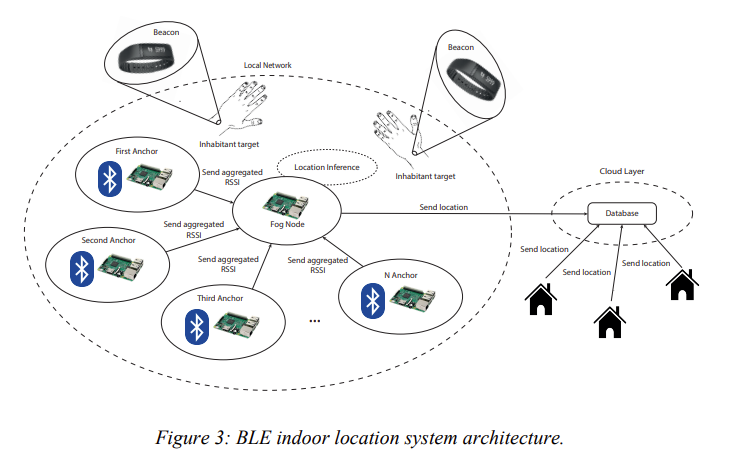
A dataset was generated with the maximum number of anchors in the indoor environment, and different configurations were then trained and tested based on this dataset. The best balance between fewer anchors and high accuracy was chosen as the optimal configuration.
This methodology was tested and optimised in a real-world scenario, in a Spanish nursing home in Alcaudete, Jaén. The experiment involved seven inhabitants in four shared double rooms. As a result of this optimisation, the inhabitants could be located in real time with an accuracy of 99.82%, using a method called the K-Nearest-Neighbour algorithm and collating the signal strength (RSSIs) in 30-second time windows.