There’s an interesting new paper titled Modeling the Trade-off between Throughput and Reliability in a Bluetooth Low Energy Connection that provides a comprehensive analysis of the performance of Bluetooth Low Energy (BLE) communication in terms of throughput and reliability under various interference conditions.
The primary objective of the study was to develop and validate mathematical models that predict the throughput and reliability of BLE connections under interference.
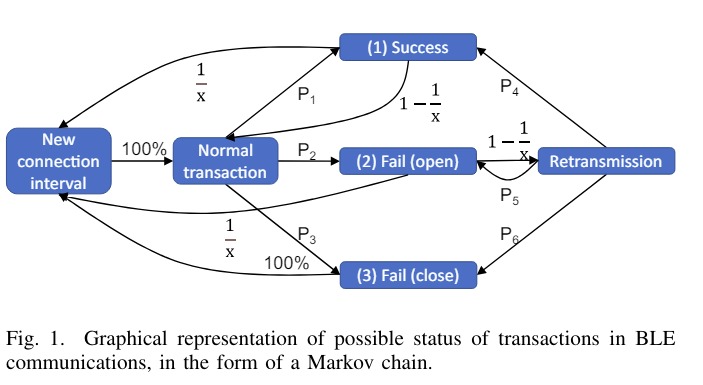
Two models were developed, a Throughput Model using a Markov chain approach to predict the throughput of BLE connections under interference, and a Reliability Model that quantified the reliability of BLE connections by considering various transmission parameters and interference levels.
The throughput model was validated through extensive practical experiments under different interference scenarios. The experiments involved varying parameters such as packet length, number of packets, and connection intervals. The results showed a close match between the theoretical predictions and the experimental data, highlighting the accuracy of the models.
As might be expected, the study found that the interference level in the environment significantly affects both throughput and reliability. Higher interference levels (higher BER) reduce both metrics.
There is a non-linear relationship between payload size and throughput. While larger payload sizes can increase throughput in low-interference environments, they significantly reduce reliability and throughput in high-interference conditions.
Increasing the connection interval improves energy efficiency but reduces throughput without affecting reliability. This suggests that connection interval adjustments can optimise energy usage without compromising communication reliability.
Bluetooth devices should be configured based on the specific interference environment they will operate in. For instance, smaller payload sizes are preferable in high-interference environments to maintain reliability.