There’s a recent paper by researchers at the Department of Management Science and Technology, Athens University of Economics and Business on An Ensemble Filter for Indoor Positioning in a Retail Store Using Bluetooth Low Energy Beacons.
The paper starts with an overview of indoor positioning techniques including trilateration, fingerprinting, dead reckoning and AI machine learning. It also provides a synposis of different technologies such as RFID, WiFi and Bluetooth.
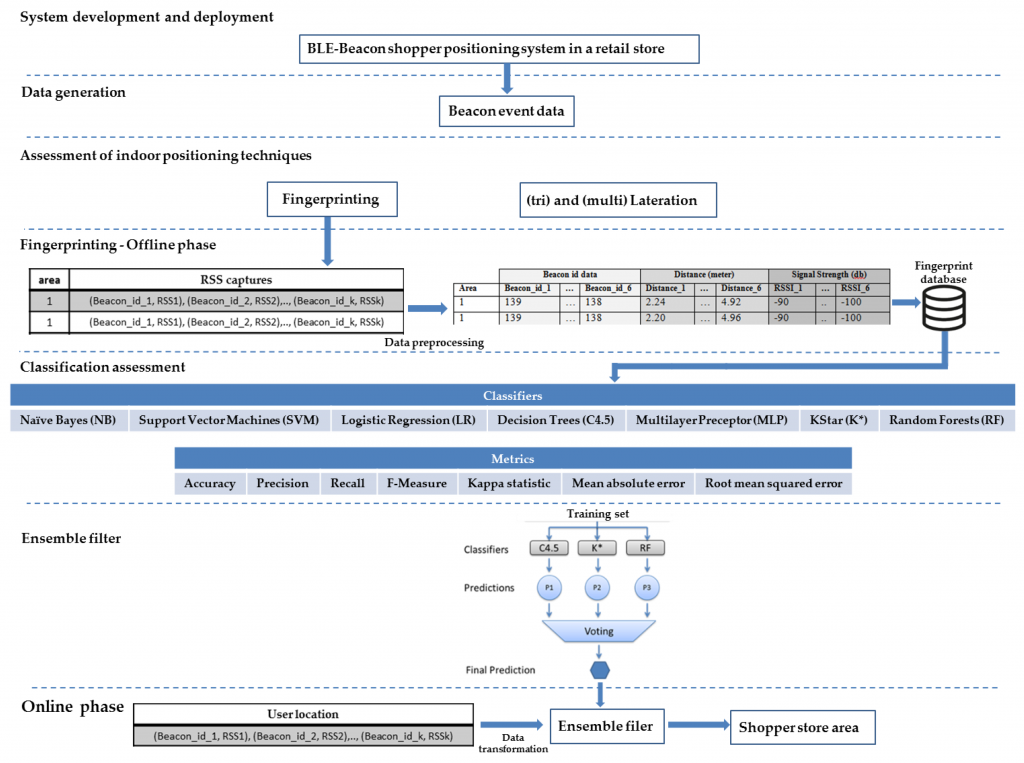
The paper explains that while fingerprinting is widely used, it faces limitations when used in dynamically changing environments. Fingerprinting requires ongoing maintenance and updating of the reference fingerprinting map that’s manually intensive and time-consuming. Fingerprinting also requires a large number of beacon reference points to perform accurate locating.
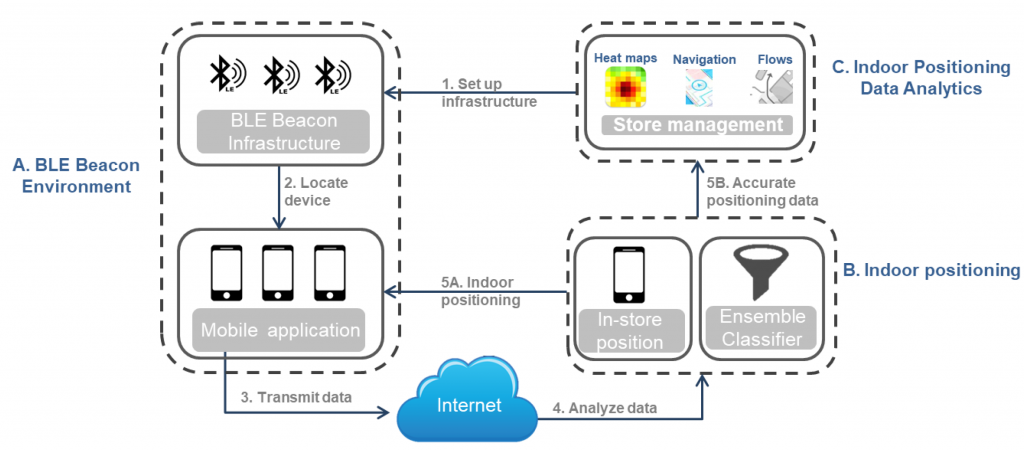
The researchers looked into positioning within a two floor (grocery) retail store. Retail stores are of of the more challenging environments as there are shoppers moving about that can affect indoor localisation
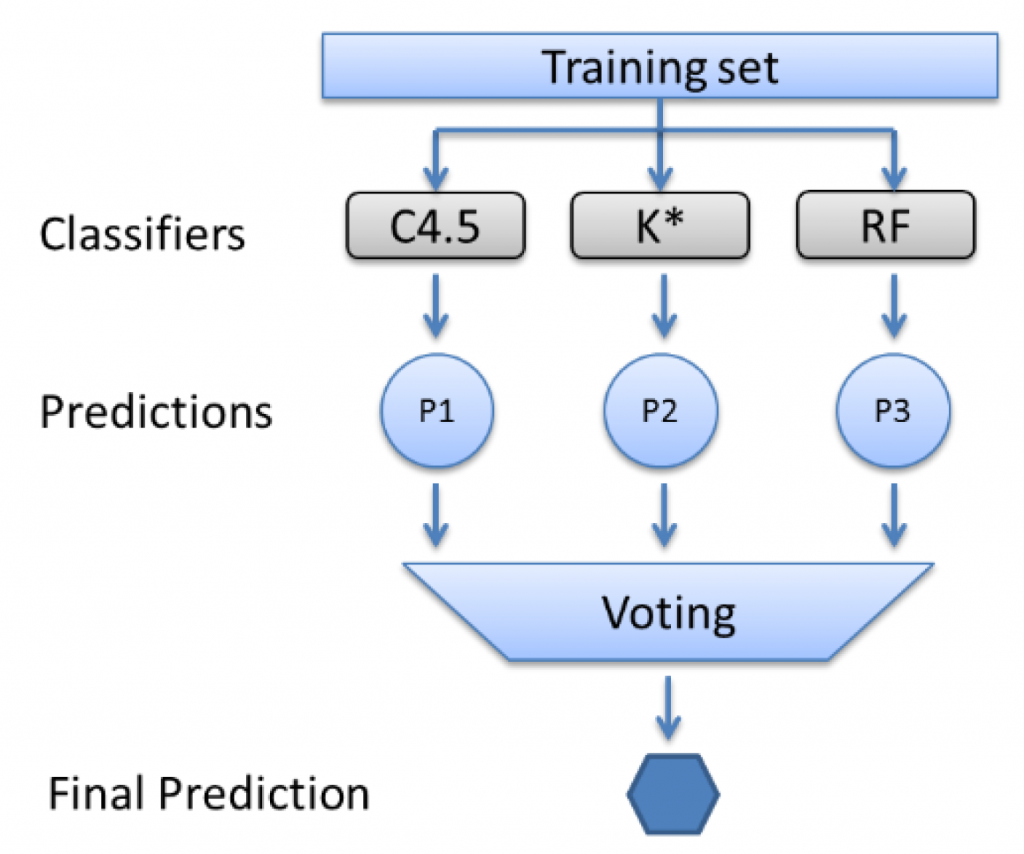
Several indoor positioning techniques were considered including fingerprinting and trilateration. The researchers implemented fingerprinting and compared it to seven established classifiers. The random forest algorithm worked the best and inspired the authors to build an ensemble classification filter with lower absolute mean and root mean squared errors.